Top 10 Leading Aws Partners to watch in 2024
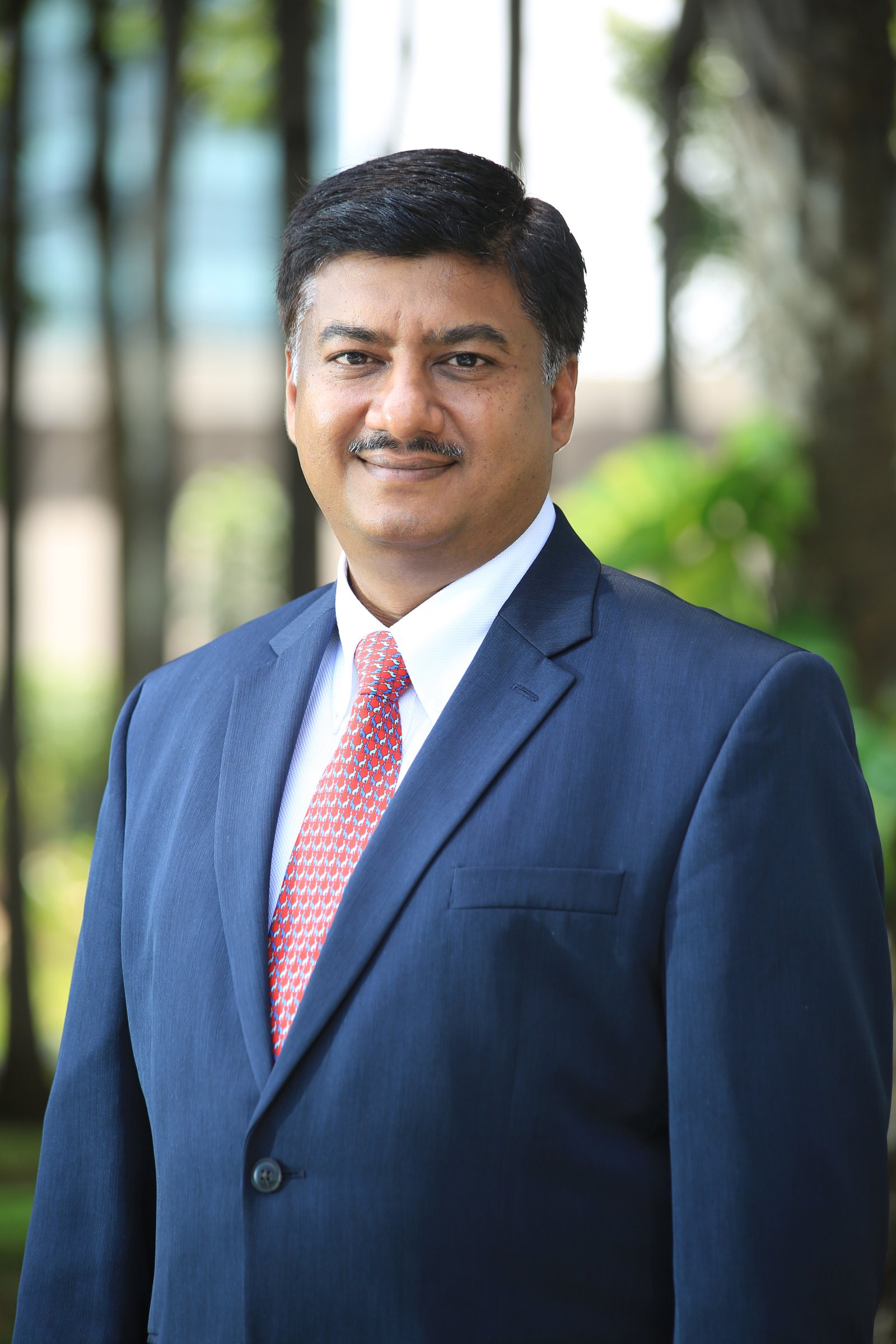
.jpg)
Specializing in full-lifecycle digital product engineering for ISVs and other tech-driven enterprises, R Systems is a premier AWS Advanced Partner. Their deep expertise in cloud, data, AI, and machine learning allows them to design and develop robust, scalable software products from chip to cloud. R Systems specializes in providing the full range of workload migration, application modernization, DevOps / DevSecOps, and cloud professional services (SRE, disaster recovery, and infrastructure monitoring & observability). They also have strong expertise in cost optimization, AI/ML-powered analytics, and generative AI-based services. They provide both cloud-native and hybrid solutions. R Systems teams work on the cutting edge of enterprise technology (both on Cloud and Digital product engineering) and have built reusable accelerators to jumpstart its engagements. R Systems, Nitesh Bansal, CEO and MD, says, “In the rapidly evolving digital landscape, enterprises are constantly seeking innovative solutions to stay ahead. As an AWS Advanced Partner with global AI/ML expertise, R Systems has been helping enterprises harness the power of Generative AI (Gen AI). Through a series of solution-led offerings, R Systems enables businesses across various verticals to unlock new business models, improve operational efficiencies, and set new benchmarks on their business KPIs (key performance indicators).” Bansal gives a detailed overview of the trends that are expected to impact R Systems as an AWS Partners Solutions in 2024 and how the company plans to leverage these trends and evolve. The Future of Enterprise Cloud and SaaS Applications The enterprise cloud landscape and the SaaS-ification of applications by new-age ISVs are evolving rapidly. Businesses will increasingly rely on AI and cloud-native solutions to drive innovation, optimize operations, and deliver exceptional customer experiences. R Systems is guiding its clients through this transformation, navigating the complexities of modern cloud usage and SaaS application development. 1. Increased Adoption of Generative AI In recent years, enthusiasm for Artificial Intelligence (AI) has surged, driving many companies to invest in AI projects. However, this initial wave, the “AI hype cycle 1.0,” faced significant challenges. Despite significant investments driven by the initial hype, approximately 80-90% of these early GenAI initiatives didn’t deliver tangible ROI, making many enterprises cautious movers. Several factors contributed to these false starts, including a lack of clear definition of desired outcomes, lack of data preparedness, a half-hearted stakeholder buy-in and underestimation of change management and training. These issues highlighted the need for a paradigm shift in how AI projects were approached. That’s when the focus shifted from merely building models to effectively applying AI in day-to-day operations. This evolution is changing the game, as companies are now more cautious about their AI investments. They are keen to see value and measurable outcomes from their efforts rather than just pouring money into projects without clear returns. “To facilitate this, R Systems provides systems and partnerships that help businesses assess their readiness to adopt Gen AI. We focus on delivering results in the shortest time-to-value, ensuring that organizations can quickly see the impact of their investments,” says Bansal One critical area of concern with AI adoption is cost. The balance between the cost of implementing AI projects and the value they deliver is crucial. While the ROI may take time to notice, it's essential to consider the long-term benefits and potential for new ideas and efficiencies that AI can bring. Reflecting on the lessons from AI hype cycle 1.0, there is a notable shift in the approach to AI projects. Many initiatives failed previously due to unclear goals and inadequate data quality. In the 2.0 phase, there's a stronger emphasis on value generation and strategic implementation. One elementary application of GenAI is the development of enterprise agents. These helpful agents can fetch contextual information quickly, saving employees time and improving their overall experience. Similarly, AI can streamline the preparation of data and reports for executives, making the process more efficient and less time-consuming. R Systems is also expanding its GenAI solutions, using AWS tools like SageMaker, Bedrock, and Retrieval-Augmented Generation (RAG). They offer tailored generative AI assessments and POCs to help enterprises implement AI-driven strategies that boost efficiency and customer engagement. Their focus is on developing domain-specific use cases to address unique industry challenges. 2. Escalating Data Security and Compliance Mandates Stricter data privacy regulations require businesses to ensure their AI solutions comply with legal standards. Secure data management and going beyond the adherence to regulations like GDPR and CCPA are crucial. R Systems’ Approach: R Systems enhances its data governance frameworks with AWS security tools such as AWS Identity and Access Management (IAM) and AWS Key Management Service (KMS). They offer services that ensure secure data management and regulatory compliance for AI models. Their emphasis on transparent data practices and ethical AI builds trust with clients. 3. Enhanced Cloud-Native Development Cloud-native development, focusing on microservices and containerization, continues to dominate as businesses seek scalable and resilient architectures. There’s a shift towards adopting a cloud-native approach, moving away from simply lifting and shifting existing infrastructure to the cloud. R Systems’ Approach: R Systems leverages AWS’s cloud-native tools, including Amazon Elastic Kubernetes Service (EKS) and AWS Fargate, to build microservices-based architectures. They develop containerized applications that offer flexibility, scalability, and rapid deployment. The Complexity of AI and ML Integration Challenge: Integrating AI and ML solutions into existing infrastructure is a common challenge for company decision-makers. The complexity of these technologies and the lack of in-house expertise often hinder effective implementation and scalability. R Systems’ Differentiation: R Systems addresses this by offering comprehensive end-to-end AI and ML services. Our approach includes detailed discovery sessions to understand specific business needs, followed by customized integration strategies. “We ensure a seamless integration process by using AWS services like SageMaker for model training and Bedrock for deployment. Additionally, we support building in-house capabilities and bridging the expertise gap through continuous support and training,” adds Bansal. Rapid Technological Advancements Challenge: Many decision-makers find it overwhelming to keep pace with rapid technological advancements in AI and ML. They struggle to stay updated on the latest tools, frameworks, and best practices. R Systems’ Differentiation: R Systems stays ahead of technological trends by maintaining a purpose-driven R&D department that explores emerging technologies and trends. The close partnership with AWS to leverage their newest services and innovations. The R System’s team’s continuous training and certification ensure they offer the latest solutions to their clients. Additionally, R Systems organizes workshops, webinars, and knowledge-sharing sessions to keep clients informed and prepared for future advancements. R Systems continues to be the trusted thought partner of its clients to demystify value from hype. Cost Management Challenge: Decision-makers are significantly concerned about managing the costs of the Cloud environment. A misconfigured Cloud environment is more of a money sink than you might imagine. There is often uncertainty about the return on investment and potential budget overruns. R Systems’ Differentiation: R Systems holds well-architected review competency and has drastically optimized and saved up to 30-40% cost savings where companies lost money due to their wrong choice of infrastructure and services. Using AWS's comprehensive cost management tools and our proprietary reference architectures, R Systems employs effective cost management strategies. The team optimizes resource usage and implements best practices for data and model management to control costs and maximize ROI. The company’s tailored solutions and expertise guide clients through cost-management complexities, ensuring impactful and cost-effective deployments. Regular financial reviews and detailed reports help clients track spending and adjust strategies as needed. Observability, Predictability & Scalability Challenge: Ensuring that the Cloud Infrastructure is right-sized and scales effectively with business growth, performing optimally under varying loads is a significant challenge. R Systems’ Differentiation: R Systems builds scalable and high-performance solutions. Leveraging AWS’s scalable infrastructure to handle increased workloads seamlessly, R Systems is also mindful of the expected loads when sizing the workloads based on data and usage patterns. Their expertise in optimizing algorithms and workflows ensures robust performance, even as data volumes and user demand grow. R Systems also implements scalable data architectures and uses auto-scaling features to adjust resources dynamically based on demand while keeping the cost in check. R Systems cloud solutions almost always implement Observability Tools, which allow real-time monitoring. This speeds up troubleshooting and reduces downtime. With more information available at all times, the Observability model also helps fine-tune resources, predict scaling needs, and optimize costs efficiently. Data Security and Compliance Challenge: With stricter data privacy regulations, businesses must ensure their AI solutions comply with legal standards. Secure data management and compliance with GDPR, CCPA, and other regulations are paramount. R Systems’ Differentiation: R Systems enhances its data governance frameworks with AWS security tools like AWS Identity and Access Management (IAM) and AWS Key Management Service (KMS). The team ensures secure data management and regulatory compliance for AI models. The focus is on transparent data practices, and ethical AI builds client trust. Regular audits and assessments ensure ongoing compliance and promptly address potential security vulnerabilities. R Systems is recognized as a global leader in technology, data, and AI/analytics services. “We provide AI-driven solutions that transform businesses using advanced technologies such as AI, ML, big data, IoT, and cloud computing. Our end-to-end support spans the entire data journey, from data management and integration to AI model development and deployment,” says Bansal. Customized AI model development, meticulous data preparation, scalable data pipelines, continuous monitoring and optimization, and a collaborative approach with clients are the keys to their success. Bansal cites two prominent case studies in detail. Case Study 1 Title: Migration from GCP and Optimization to Scenario: The customer is a US-based transportation services provider hosting a large GCP infrastructure. They host multiple applications using Python, Node.js, and GoLang, and Analytics for their Mobile applications (iOS and Android). The Web and Mobile apps were used to book rides and track vehicles in real-time. Engagement: The team assessed the application environment and drafted a plan to enable switchover with minimal downtime. A similar environment was set up in AWS, and then the workloads migrated over. Containerized the applications and implemented performance monitoring with horizontal pod scaling to handle changing user loads. The application team separately worked on applications and databases to optimize them. Implemented monitoring and alerting mechanisms across the infrastructure and provided Site Reliability Engineering support. Impact: R Systems reduced costs by almost 60% and increased performance by another 40% simply by optimizing the existing infrastructure, database queries, and right-sizing the virtual hardware. SRE support ensured that when problems occurred, support was available on-call 24/7. Case Study 2: Title: Code Repository migration from SVN to AWS CodeCommit and CI/CD Pipeline setup, with Code Analysis Scenario: The customer had a sizable code repository hosted on-prem using Subversion (SVN). The project team reported performance issues and a lack of automated deployment. Engagement: The team successfully migrated the entire project codebase to AWS CodeCommit and set up branching and tagging strategies. Implemented SonarQube for Code Analysis. Defined separate environments for Dev, QA, Stage, and Prod. Implemented a container-based approach to deploy the application. Set up CI/CD pipeline with fully automated testing. Implemented Blue-Green Deployment strategy with off-site DR. Impact: The customer was pleased with the new environment and reported significant improvements in their dev processes. Implementing Code Analysis tools also helped improve code quality, and fewer bugs were reported in Automated QA. A Blue-Green deployment strategy also helped ensure that Business impact was minimal in case the build failed in Production. R Systems has gradually established a strong position in the technology, data, and AI/analytics services industry. These elements give them a competitive edge and make them a noteworthy AWS partner. Speaking about the future, Bansal says, “The future of R Systems is marked by strategic expansion and continuous innovation. We are committed to extending our geographic and industry footprint, ensuring that we can deliver our cutting-edge solutions to a broader range of clients across the globe. Our focus on enhancing our platform with the latest AI and ML technologies will keep us at the forefront of the digital transformation landscape.”