Top 10 Fastest Growing Quantum Computing Companies To Watch In 2023
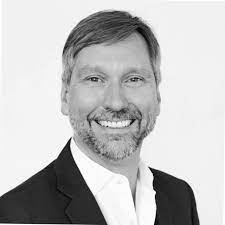
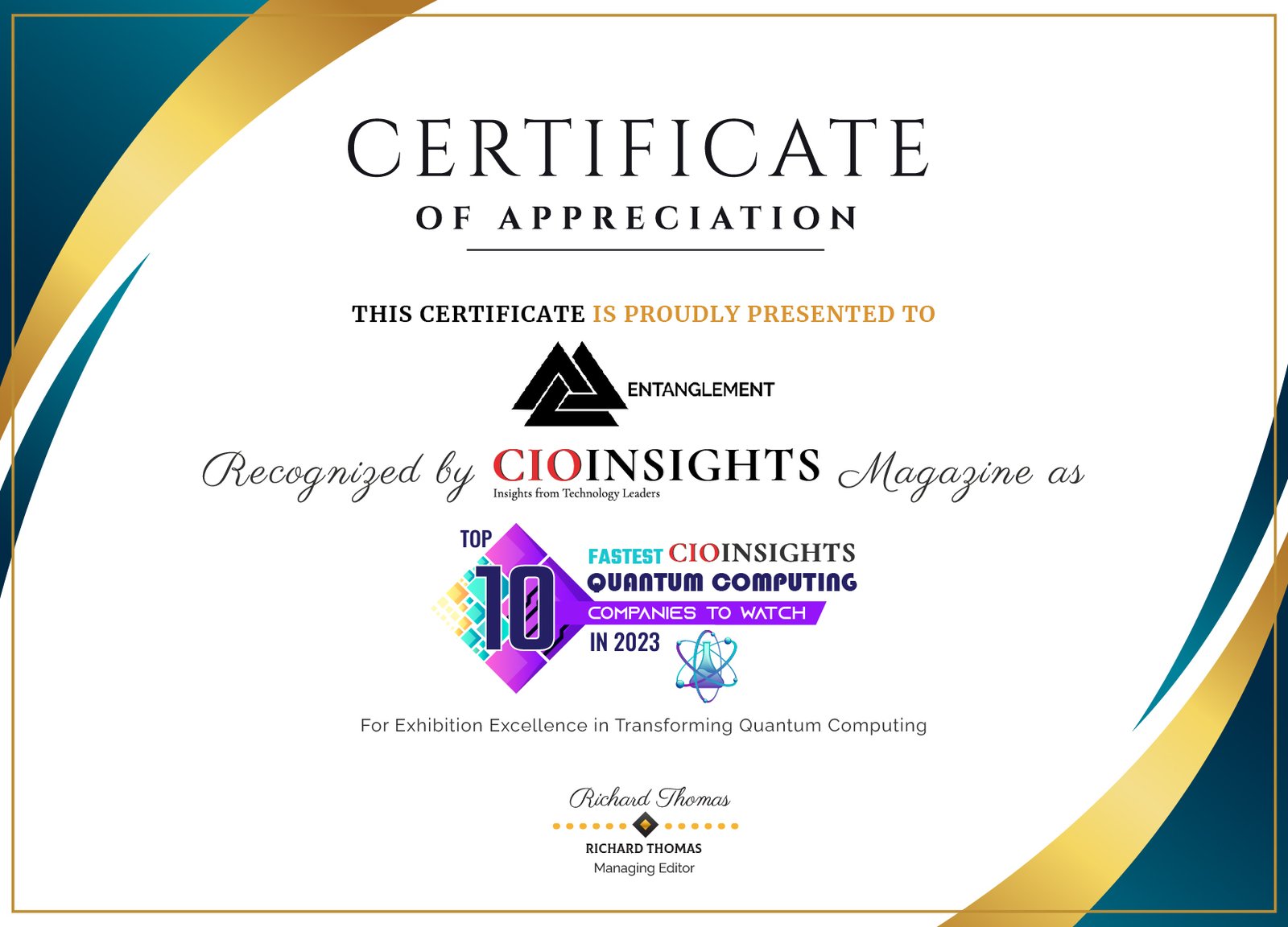
Entanglement.ai: Bridging the Future of Quantum Computing and Artificial Intelligence The dawn of quantum computing has ushered in a new era of computation, promising unprecedented processing power and capabilities that were once thought to be confined to the realm of science fiction. At the intersection of this revolutionary technology and the rapidly evolving field of artificial intelligence (AI) lies a groundbreaking concept: Entanglement.ai. In this article, we delve into the fascinating convergence of quantum computing and AI, exploring the concept of Entanglement.ai and its potential to reshape industries, drive innovation, and unlock previously unimaginable possibilities. Quantum Computing: A Glimpse into the Subatomic Before we embark on the journey of understanding Entanglement.ai, it is essential to grasp the fundamentals of quantum computing. Unlike classical computers that use bits as the smallest unit of data, quantum computers employ quantum bits or qubits. These qubits exist in a superposition, representing multiple states simultaneously, and can be entangled, a phenomenon where the state of one qubit becomes inherently linked to the state of another, regardless of the physical distance between them. This concept of entanglement forms the bedrock of quantum computing's exponential computational power. While classical computers process information sequentially, quantum computers harness the power of entanglement to perform parallel computations, enabling them to solve complex problems with astonishing speed. Quantum computing has the potential to revolutionize various domains, from cryptography and optimization to drug discovery and material science. The Rise of Artificial Intelligence In parallel with the advancements in quantum computing, the field of AI has been experiencing a renaissance of its own. Machine learning, a subset of AI, has led to remarkable achievements in image recognition, natural language processing, and autonomous systems. Traditional machine learning algorithms, however, face limitations when it comes to processing vast amounts of unstructured data and tackling intricate problems that arise in fields like genomics, climate modeling, and financial analytics. Enter deep learning, a subfield of machine learning inspired by the structure and function of the human brain. Deep neural networks have demonstrated unparalleled performance in handling complex tasks, making them the driving force behind many AI breakthroughs. Yet, even these sophisticated models are not immune to the constraints imposed by the classical computing hardware on which they run. Entanglement.ai: Uniting Quantum Computing and AI Entanglement.ai emerges as a revolutionary concept that seeks to bridge the gap between quantum computing's potential and the demands of advanced AI algorithms. At its core, Entanglement.ai leverages the phenomenon of entanglement to enhance the processing capabilities of AI systems. By exploiting the parallelism inherent in quantum entanglement, Entanglement.ai aims to accelerate training and inference in AI models, enabling them to process and analyze vast datasets in a fraction of the time it takes traditional classical systems. One of the key advantages of Entanglement.ai lies in its potential to tackle combinatorial optimization problems, which underpin various real-world challenges. Examples include optimizing supply chains, portfolio management, and resource allocation. These problems often involve a vast number of variables and constraints, making them computationally intensive for classical systems. Entanglement.ai, with its ability to explore multiple solutions simultaneously, could revolutionize these domains by rapidly identifying optimal or near-optimal solutions. Quantum-Inspired Machine Learning vs. Entanglement.ai It's important to distinguish between quantum-inspired machine learning (QML) and Entanglement.ai. QML involves using classical hardware to simulate quantum processes and explore the potential speedup that quantum algorithms might offer. While QML has shown promising results in some cases, it is still limited by the capabilities of classical hardware. On the other hand, Entanglement.ai goes beyond simulation and aims to harness the true power of entanglement by leveraging quantum computing resources. This crucial distinction is what sets Entanglement.ai on a trajectory to potentially achieve groundbreaking advancements in AI that were previously unattainable. Applications of Entanglement.ai The convergence of quantum computing and AI through Entanglement.ai opens up a myriad of possibilities across various industries. Drug Discovery and Genomics: The pharmaceutical industry could benefit immensely from the accelerated computational power of Entanglement.ai. Simulating molecular interactions, identifying potential drug candidates, and understanding complex genetic mechanisms could all be expedited, leading to faster drug development and more personalized medicine. Financial Modeling: In the world of finance, predicting market trends and optimizing trading strategies are incredibly complex tasks. Entanglement.ai could process vast amounts of financial data to provide more accurate predictions and refine trading algorithms, potentially minimizing risks and maximizing returns. Climate Modeling: Climate scientists grapple with the complexities of Earth's systems when making predictions about climate change and its impact. Entanglement.ai could enable more precise and rapid analysis of climate models, helping researchers make informed decisions to mitigate environmental challenges. Artificial General Intelligence (AGI): The pursuit of AGI, where machines possess human-like cognitive abilities, could be significantly accelerated by Entanglement.ai. Training complex AGI models currently demands colossal computational resources and time. Entanglement.ai could slash these requirements, bringing us closer to the realization of AGI. Challenges and Considerations While the promise of Entanglement.ai is tantalizing, several challenges and considerations need to be addressed: Quantum Hardware Limitations: Quantum hardware is still in its infancy, prone to errors due to decoherence and noise. Entanglement.ai's effectiveness is intricately tied to the quality and reliability of quantum processors. Algorithmic Development: Developing algorithms that can effectively leverage the power of entanglement while minimizing error amplification remains a significant challenge. The field of quantum error correction is essential in this regard. Hybrid Approaches: Hybrid quantum-classical systems, where quantum processors assist classical computations, might be a pragmatic approach in the near term. Designing seamless integration between classical and quantum components is a non-trivial task. Ethical Implications: As with any powerful technology, Entanglement.ai raises ethical considerations. Ensuring the responsible and secure use of this technology is paramount to prevent unintended consequences. Entanglement.ai stands at the crossroads of quantum computing and AI, offering a glimpse into a future where computation reaches unparalleled heights. As quantum computing continues to mature and quantum hardware becomes more stable, the potential of Entanglement.ai to revolutionize industries and reshape our world becomes increasingly feasible. The convergence of these two transformative technologies is not just a technological milestone but a paradigm shift in our approach to solving complex problems. Entanglement.ai is a testament to human ingenuity, pushing the boundaries of what is possible and propelling us into a future where the impossible becomes routine, and the unimaginable becomes reality. As we venture deeper into the uncharted territory of quantum-powered AI, we are poised to unlock a new era of innovation and discovery, forever altering the landscape of computing and artificial intelligence.