The Future of Privacy and Quantum
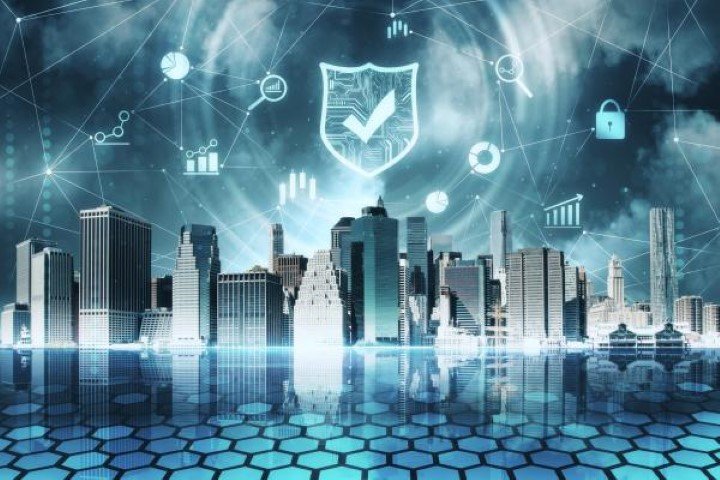
Safeguarding data protection is not so hard.
It only requires a real understanding of the ethically and legally justifiable purpose of the envisaged processing of personal data, of the relevant context of the nature of the processing, and of the data involved, of the related risks for the individuals whose data is processed as well as for the organisation for (not) processing the data, and adequate and timely selection of the relevant risk mitigating measures, based on accepted norms and standards.
Two years ago I created this infographic (Source) to compare, what we could frame as a 'classic context' for processing, compared to today's context:
In other words, the basic principles of data protection are valid in different contexts - all it requires us to do is to define, for these new contexts, what is considered just, fair and ethically desired. This is something we collectively find hard. We now also are compelled to understand the legalities and technical details of Machine Learning, of processing not datapoints or linking datasets, but combining data lakes and have algorithms visit these.
There are two weaknesses, I see, in our collective approach, that combined have a crippling effect on responsible innovation. We look at risks primarily from a legal point of view, and at the same time we do not exactly understand technically how the processing is done (not only in cases of unsupervised learning) and how the new capabilities in the nature of processing (quantum computing) and the nature of data (data lakes) fundamentally change the context of our accountability and responsibilities.
Our collective incapability to assess risk
In a recent paper by David Rosenthal: "Die Tücken spontaner Datenschutzbeurteilungen und was sich dagegen tun lässt" (Source) we learn (page 10, ibidem), based on empirical evidence, that risk assessment currently is far from trustworthy - ask different experts and they will attribute different values to the components that constitute a risk: likelihood (Eintrittswahrscheinlichkeit (probability)) and impact (Schwere des möglichen Schadens (severity)):
In my experience as a DPO I see this every day in play. The differences in risk assessment are sometimes rhetorically framed as a matter of risk appetite, usually where the risk aversive people frame people that have a different risk assessment of being irresponsible for qualifying what they would rather like to see as a high risk ('we put it on "high" just to be sure'). In most cases the risk aversive people look at the impact side of the equation and not at the likelihood aspect. A high impact and a zero likelihood results in a zero risk though. And risk appetite is a decision to be made after the risk itself has been identified.
This troublesome observation about our collective inability to identify, is alike cases, the same risk level, causes a crippling effect in the areas where these risk assessments usually are made - in the field of research and innovation. Inadequate risk assessment is blocking responsible research and innovation.
There are, to my knowledge, not so many multi stakeholder debates yet, dedicated on establishing criteria for determining "likelihood / probability" and "impact/severity". If we would do so, we would also take into consideration the mitigating measures that, for instance, lower the "likelihood / probability" of a certain event to happen. For instance, in our Data Transfer Impact Assessments, when determining data transfers to third countries, where encryption of communication, combined with encryption of data in transfer and in rest (with the controller being the only one with access to the encryption keys), and for instance, the use of zero knowledge services, do actually limit the "likelihood / probability" of illegal further processings of the controller's data by the data processor in the third country.
This is worrying for many reasons. I would like to point out two reasons. Immature risk assessment cripples and frustrates research and innovation. And it creates unjustifiable risk shortcuts - there will always be organizations that see the business case of consciously and purposely underestimating risks, and thus act as a magnet for risky processings. The 'Risk Havens' (see: "Tax Haven").
Contemplating the future of privacy, the elephant in the room.
How well are we, collectively, doing, from a GDPR point of view, with 'classic context' processings. So the 'easy cases' where an employee on behalf of an organization processes data of a data subject, based on consent of the data subject. Are all aspects of the processing communicated clearly and in plain language communicated transparently to the data subject? Is this still the case when cloud services are used, third parties from third countries and algorithms are used?
Elephant: Quantum Computing. IBM's Eagle Quantum Computer.
You may have missed it, but quantum computing is commercially available and expected by IBM and the Boston Consulting Group to create a $3B+ revenue in Financial Services, Drug Design and Materials Design (Source):
IBM has, in 2021, reached the end of what they call their IBM Quantum System 1, with their Eagle (a 127-qubit quantum processor), and are now focusing on their System Two:
The current available IBM Quantum Computing capabilities becomes clear if you let sink in what IBM has achieved with bringing quantum computing to the cloud and their approach of "Quantum Serverless", where the scientist can just focus on code, as it were, on classical computation or high performance computing, and where the integration of classical computation and quantum computing is delivered as a service, providing a claimed 120 x speedup.
In a recent Nature article, Davide Castelvecchi warns us with his: Preparing for Q-Day. The quantum-computer revolution could give hackers superpowers. New encryption algorithms will keep them at bay. He point at evidence provided by Peter Shor that quantum computing is expected to to break public-key cryptosystems such as RSA. Shor’s quantum algorithm could also efficiently break an elliptic-curve key.
"In 2015, the NSA’s unusually candid admission that quantum computers were a serious risk to privacy made people in policy circles pay attention to the threat of Q-day. “NSA doesn’t often talk about crypto publicly, so people noticed,” said NIST mathematician Dustin Moody in a talk at a cryptography conference last year."
This is begging the question: who, other than NIST is doing work in the security and privacy implications of quantum computing? We see developments where the potential of ML/AI and quantum computing are explored:
To be sure: my goal would be to understand the nature of these capabilities, and to think of ways that their use in privacy by design and security by design are ensured. Not as a new way to cripple responsible research and innovation and later on, have a ritual bandwagon discussion on how quantum computing should have been human centered