The Convergence of Artificial Intelligence (AI) and Data Analytics and its implications for Healthcare Providers and Payers
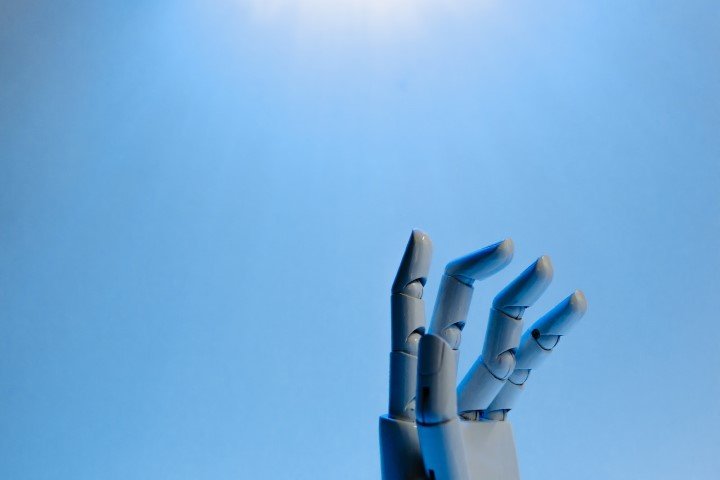
In my previous blogpost, ‘How will Artificial Intelligence (AI) and Analytics impact Healthcare Providers, Payers, Pharma, Medical Devices and Diagnostics’ published prior to the HIMSS 2019 National Conference, I had predicted the convergence of AI (comprising machine learning, natural language processing (NLP), deep learning and robotics) and Analytics to drive measurable improvements in business, clinical and patient outcomes, which received positive feedback and support from my global readership.
I must candidly admit I am truly excited and humbled to see that the findings from a recent research survey ‘Data Analytics in Healthcare’ by HIMSS Media totally validates this prognosis. The research report also highlights the key areas of investments in AI and Analytics by healthcare providers and payers in 2020-21, as well as their priority areas of value, which I will articulate in this post.
Leaders in healthcare see tremendous potential in AI and Analytics to deliver on the promise of higher quality care at a lower cost by empowering their executives, business leaders, clinicians and nurses by harnessing the power of predictive and prescriptive analytics. Many healthcare organizations are seeking to harness the vast potential of artificial intelligence (AI) and its four components — machine learning (ML), natural language processing (NLP), deep learning, and robotics — to transform their clinical and business processes. They seek to apply these advanced technologies to make sense of an ever-increasing ‘Tsunami’ of structured and unstructured data, and to automate iterative operations that previously required manual processing.
How are innovative Healthcare Organizations planning and deploying their AI solutions?
HIMSS Media’s January 2020 research report ‘Data Analytics in Healthcare’ surveyed the convergence of data analytics and AI among payers and providers.
Here are the key findings from the research survey:
Cautious Optimism re: AI from Healthcare Leaders
The research surveyed leaders employed in IT/cybersecurity, informatics, clinical, and business-related roles at U.S. hospitals and health systems and identified 4 key findings from the data:
- AI is a priority for many organizations, with 3 in 4 respondents agreeing on specific areas for immediate implementation.
- Data analytics confidence decreases as complexity increases.
- Barriers to success with complex data analytics are multiple and diverse.
- The distribution of data analytics resources continues to tilt slightly toward clinical use cases at this time.
Despite that commitment to AI-infused analytics, only 56% of those surveyed rated their current descriptive analytics initiatives as extremely or very effective, and only 4 in 10 do so for predictive or prescriptive analytics. Clearly, it is a case of excitement tempered by a mix of caution and frustration.
Per my experience engaging with real-world practitioners and industry thought leaders at the recent AI4Healthcare Conference in NYC articulated in this recent blogpost, ‘Will AI augment Hospital people, processes and systems, in the foreseeable future?’ there is significant concern re: the lack of transparency manifested in a “Black Box approach” from many point solution vendors in the AI arena. Clinical leaders are rightfully apprehensive re: leveraging the recommendations or acting upon analyses from algorithms that have not been validated by them or their experts, with life impacting implications.
In addition to this “black box” anxiety, the research turned up a number of other barriers to adoption, as illustrated in figure 1 below.
Chief among these are:
- Difficulty working with vast amounts of unstructured data
- Difficulty capturing, accessing, and integrating data from disparate sources, whether large databases, devices, or mobile apps; and
- An inability to up-skill employees to accomplish more complex data analytics requirements.
All three of these barriers reflect a mismatch in the complexity of the tasks with the skill base and resources available to deploy AI-based solutions.